Healthcare.AI™
Expanding the Use of AI in Healthcare
What is Healthcare.AI?
Expanding the Use of AI in Healthcare
Healthcare.AI™ from Health Catalyst
Use links below to jump to the following sections
Healthcare leaders face an unprecedented list of increasingly critical issues across revenue, cost, and quality. These leaders are turning to artificial intelligence as the key to better decision-making, believing it will deliver faster turnaround of insights with small margins for error. While these attempts may even incorporate augmented intelligence (AI), which goes beyond artificial intelligence by enabling human decision makers to make the best choices, they often fall short.
As a result, many business and analytics leaders are trying to integrate augmented intelligence (AI) into their analytic processes to better address these critical issues. Too often, these efforts have been less than successful. Leaders have struggled to integrate AI into current tools, integrate or change workflows, and demonstrate a positive impact of AI.
The first release of Healthcare.AI by Health Catalyst focused primarily on point of care or service predictive model use cases. However, this approach was often ineffective when applied without expert guidance (self-service), and the approach was also too narrow in scope, limiting the effective use of AI in healthcare.
The Healthcare.AI offering from Health Catalyst is an improved, transformational suite of products and expert services that address this wider array of critical business issues. Healthcare.AI dramatically broadens the use and use cases for effective AI within your organization.
Healthcare.AI dramatically expands the use and use cases for effective AI within your organization.
Why Is AI not living up to expectations In Healthcare analytics?
Three common challenges prevent successful AI integration at health systems:
Challenge 1: Integrating AI into Current Tools
Ideally, analysts want AI capabilities integrated into their existing workflows and business intelligence (BI) tools. However, most AI tools are standalone applications requiring analysts to learn and adopt yet another program, language, or integration point. Either approach limits the adoption of AI into key analytics workflows.
Challenge 2: Integrating AI Expertise Within the Organization
Effective AI integration requires data science expertise, and the leaders who attempt to fill this expertise gap find it challenging for myriad reasons:
- Health systems simply cannot adequately fund data science resources.
- Even with in-house data science talent, scaling that expertise to the broader analytics teams is difficult.
- Teaching data science concepts and skills to existing teams is a slow, expensive, and unsustainable process.
Challenge 3: Demonstrating the Positive Impact of AI
When healthcare organizations have managed to implement predictive models, they struggle to demonstrate the impact and engender trust throughout the organization. Some of the most common challenges include:
- Limitations of “black box” software: Most traditional artificial intelligence solutions are “black boxes,” which make it impossible to validate, modify, or optimize the analysis. Leaders are left wondering if these programs are working or how to improve them.
- Narrow focus on predictive analytics: Many of these solutions narrowly focus on predictive analytics, limiting any potential impact. While predictive modeling is an important use case, the opportunities for AI support are enterprise wide, including for critical decisions.
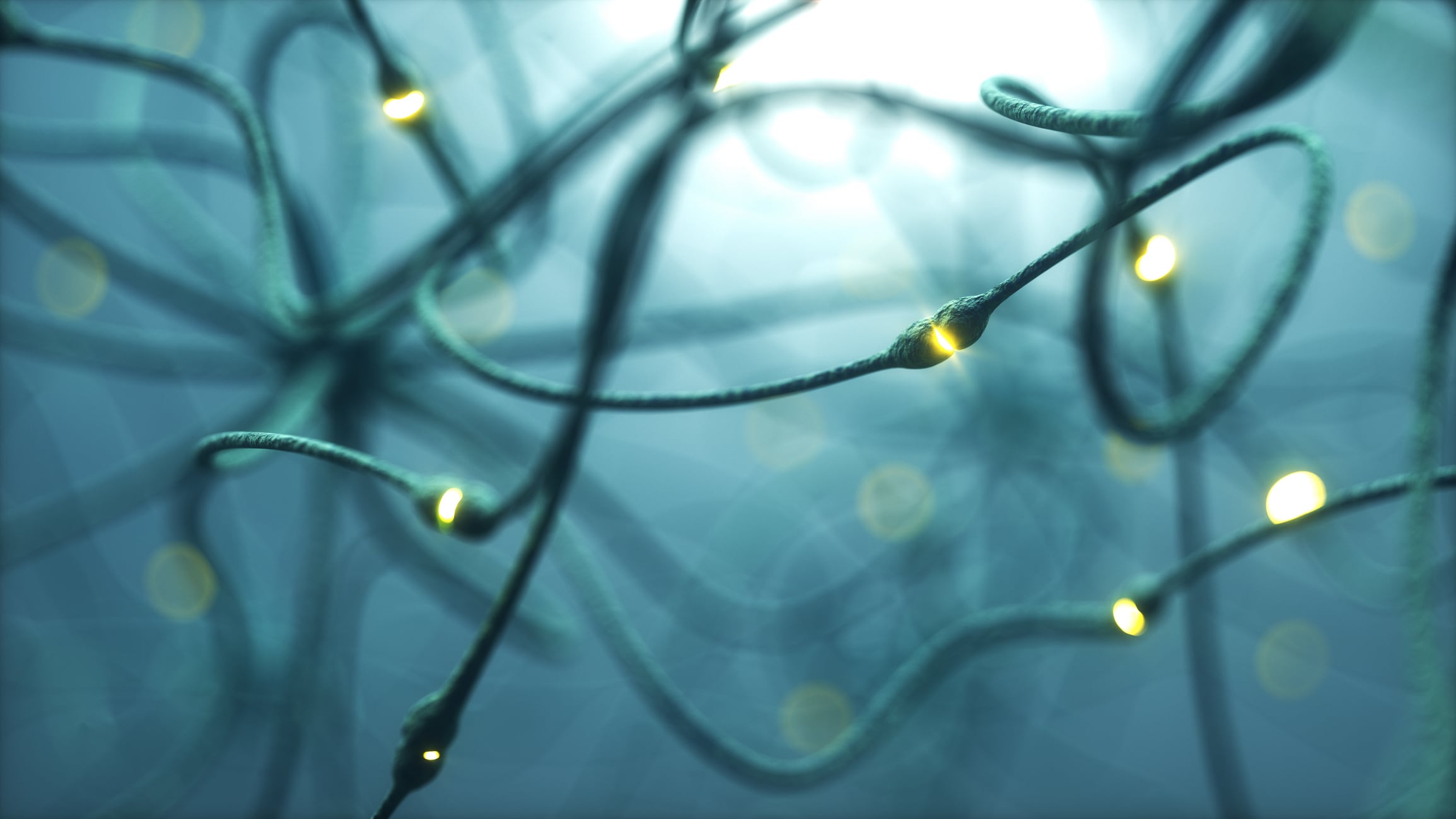
How Healthcare.AI Solves These Issues
A transformative and improved product suite and set of expert services, Healthcare.AI by Health Catalyst, dramatically broadens effective AI use throughout healthcare organizations.
The offering is optimized to address five levels of analytics AI use cases as laid out in the Healthcare.AI Framework™:
Level 1: Analytics Integration – Healthcare.AI Product Suite
Our Healthcare.AI Product Suite expands the easy use of self-service AI into your mainstream analytics.
Provides easy one-button-click AI access into existing business intelligence (BI) tools and applications.
The Healthcare.AI Product Suite easily integrates into your existing BI tools and Health Catalyst applications. Once installed, it can be up and running and creating insights in minutes. The Healthcare.AI Product Suite easily integrates into:
- Your existing business intelligence tools (Qlik, PowerBI, Tableau, Leading Wisely).
- More than 100 Health Catalyst analytics accelerators.
- Your existing Health Catalyst software product lines (more coming in 2021).
Embeds a blend of well-established and cutting-edge statistical and machine learning techniques into every product suite module.
We start with embedding proven statistical methods successfully used in our years of data-driven healthcare experience.
Then we add the latest AI technology to improve the existing analytics module capability.
Our embedded approach makes it easier for healthcare analysts of all types and backgrounds to understand and use advanced statistical and AI features in their most common use cases, within their existing business intelligence workflows.
Helps analysts produce the higher quality and dramatically faster insights needed to support your business-critical issues.
The embedded rigor means that your analytics insights are more accurate, precise, and consistent than before. You do not have to wait months or even years for additional training or hands-on experience to give your analysts an accelerated AI boost.
This embedded approach dramatically increases the speed to deliver analytic insights from months or weeks to minutes or seconds. It also automates analytics tasks that currently take time in external tools and languages and introduces advanced AI that helps identify trends much faster than more traditional approaches.
Levels 2-5: AI Expertise
Our Healthcare.AI Expert Services help significantly expand and improve your success rates for advanced AI use cases.
We help you choose, build, or optimize from hundreds of predictive models (Levels 2,3).
Transactional predictive analytics are the most common use of AI in healthcare analytics. Because of this, predictive models and self-service predictive model building engines have proliferated to meet a wide variety of needs. However, without expert guidance in selecting or tailoring these tools, many pitfalls exist preventing optimal deployment in local healthcare decisions.
To overcome that challenge, our Healthcare.AI offering provides expert guidance to help you pick, tailor, or build predictive models optimized for your organization and business needs.
- We start with hundreds of pre-defined predictive models from which to choose.
- We help choose, tailor, or build the optimal predictive model for your situation.
- We help you validate the predictive model and optimize the impact within your organization.
Our approach ensures that you get the best set of predictive models with the greatest likelihood of demonstrating results.
We help you use AI for advanced use cases beyond predictive analytics (Levels 4,5).
AI in healthcare has too often been limited to predictive analytics. While predictive modeling is important, there is a much greater set of uses and opportunities for AI in healthcare.
We provide expert guidance to help you identify and use AI for a wider variety of use cases and advanced business decision-making situations, including major categories such as:
- Retrospective comparisons for system-wide improvement optimization.
- Prescriptive optimization to plan for your most efficient and effective go-forward improvement projects.
We can help you start, expand, guide, or supplement your data science talent.
Experienced healthcare data scientists can be hard to find, recruit, and retain. Many organizations also struggle to integrate their data science talent into their broader analytics efforts.
We provide expert healthcare data science talent. We can help you with the right level of guidance—from supporting a “light touch” data science project to embedding our data science resources to help you with longer-term efforts (a process we have been refining since the inception of Health Catalyst).
Our Healthcare.AI Approach
Our entire Healthcare.AI approach is uniquely open, transparent, and easy, unlike most AI “black box” product solutions.
Typical AI product solutions deliver results without giving leaders and users a clear understanding of how results are derived. This approach is often called black box because the methods for delivering the results are largely unknown to the users.
Black box AI solutions are challenging because:
- Their results and recommendations are hard to explain or validate.
- Inaccurate results are hard to diagnose or modify when they don’t appear to be working.
This opacity makes it difficult for business leaders or clinicians to trust AI-driven recommendations, rendering the results either ineffective or at times rejected.
Truly effective AI solutions—the algorithms, people, and workflow—must first be coupled together to solve specific problems and then tuned over time for optimal results. Easy and open transparency becomes critical for everyone involved—those leading change, the analyst, and the end user—to optimize and trust the results.
We specifically designed our approach to be open and transparent.
Our products are built to easily integrate into your analytics workflow, giving you the same transparency that you have learned to trust from your BI tools.
Our tools and services help you build trust and lead change through driving engagement and fitting the data, methods, and algorithms to your specific situation.
These approaches help you significantly increase the use and adoption of AI in your organization because you can build the trust and confidence of downstream users in the AI-influenced recommendations.
Healthcare.AI Framework™
Expanding the Use of AI in Healthcare
Healthcare.AI Framework™
Use links below to jump to the following sections
The Healthcare.AI Framework identifies five distinct categories of augmented intelligence (AI) usage in healthcare. Each level represents a set of questions paired with a set of analytics and AI technique.
Although the levels are not necessarily sequential, they do represent levels of analytics complexity, with the lowest level (Level 1: Analytics Integration) consisting of the most common and widely used set of analytics use cases, and the highest level (Level 5: Prescriptive Optimization) demonstrating the most advanced categories of AI analytics use cases. This framework both categorizes and expands the potential use cases for AI within healthcare. To date, most healthcare organizations think of Level 2: Predictive Analytics as the primary use for AI.
The Healthcare.AI Framework significantly expands the potential use of AI in healthcare to a much broader and wider variety of use cases. This framework significantly broadens the use and use cases for which AI can be applied in healthcare. It significantly broadens:
- The number of potential AI users by applying AI to the most common and basic analytics use cases in Level 1.
- The scope of potential AI use cases by identifying complex, system-wide, business-critical issues
Questions
Capability
Solution
What is our trend and timeframe to show improvement?
- What is our past readmission rate and where is it going?
- How long will it take to demonstrate improvement?
Level 1: Analytics Integration
- Statistical Process Control, Time Series Outlier Detection, Forecast, Power Analysis, Forest Plots, COVID 19, Multi-dimensional Time Series Clustering, Peer Finding.
Help me choose, tailor, or build a predictive model for…
- Which patients are at greatest risk for readmission?
- Which patients will not complete their post-discharge follow-up visit?
Level 2: Choose/Build Predictive Models
- Hundreds of pre-defined or tailored model options.
- Model efficiency.
When and how should we deploy a predictive model?
- Does this readmission prediction model make sense?
- At what risk level should a case manager reach out to a patient?
Level 3: Optimizing Predictive Models
- Functional Model Understanding, Operational Model Understanding, Contextual Model Understanding.
Did we improve over time or versus peers?
- Did Hospital A achieve better readmission performance over time?
- Does Hospital A have lower readmission than Hospital B?
Level 4: Retrospective Comparison
- Statistical Modeling, Group Differences, Confounding, Causation, Sensitivity Analysis.
How might we improve in the future?
- How can I find the best way to follow up with patients after discharge?
Level 5: Prescriptive Optimization
- Problem Refinement, Learning Design, Simulation.
Healthcare.AI Offering From Health Catalyst
Expanding the Use of AI in Healthcare
The Healthcare.AI™ Offering
Use links below to jump to the following sections
Healthcare.AI Product Suite (Level 1)
Expand the easy use of self-service augmented intelligence (AI) in your mainstream analytics.
- Provides easy one-button AI access into existing BI tools and applications.
- Embeds statistical, AI, and healthcare rigor into every module.
- Helps analysts produce higher quality and dramatically faster insights.
Healthcare.AI Expert Services (Levels 2-5)
Significantly expand and improve success rates for more advanced AI use cases.
- Choose, build, optimize from hundreds of pre-defined predictive models.
- Use AI for advanced use cases beyond predictive analytics.
- Start, expand, guide, or support your data science talent.
Healthcare.AI is the fastest and easiest way to dramatically increase the effective use of AI throughout a healthcare organization.